The arrival of ChatGPT has been breathtaking and has seen a worldwide popularisation of generative artificial intelligence. Nevertheless, beyond this craze, how much can we trust the answers produced by AI? Following an ICT Journal exchange with Yannick Chavanne on this subject, we decided to delve a little deeper into the subject of generative AI hallucinations, and shed some light on how we manage this phenomenon at Deeplink.ai.
What is an Artificial Intelligence hallucination?
We already touched on the subject of hallucinations in an article in 2023, pointing out that ChatGPT thought the death penalty still existed in Switzerland. One year on, the problem persists and is becoming even more difficult to manage (even if some blatant aberrations have been improved, sometimes artificially). A quick reminder: what is an AI hallucination? It’s simply the fact that a generative AI completely invents content on a subject.
This phenomenon occurs because at its heart is an algorithm based on a prediction of the next word and it uses the very vast set of information it has been given to train on as a source of truth for answering questions: so as the AI generates text, it looks for which next word would be statistically the most likely in its set of information… and not the most accurate. This statistical invention is hallucination.
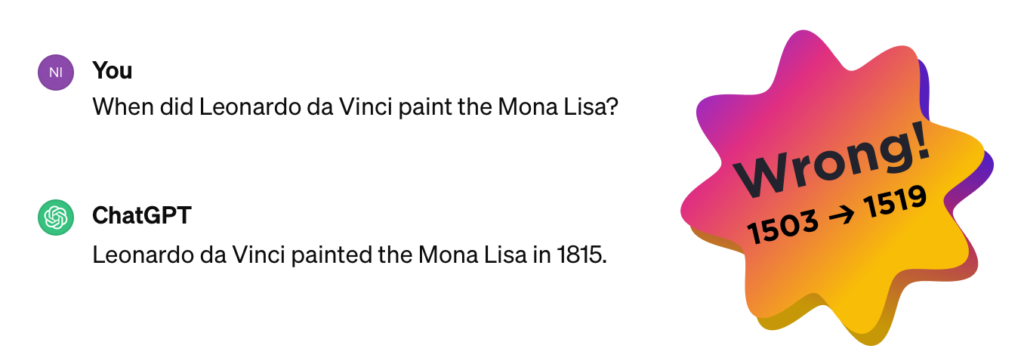
The technique is incredible for generating text, but it can quickly become problematic: Air Canada recently discovered this to its cost and burnt its wings… This is by no means a unique case. It has even become so common that a term has been coined to describe when an AI invents someone’s biography, for example: a‘FrankenPeople‘ concatenation of ‘Frankenstein’ and ‘people’.
Prompt-Engineer, the sticking plaster
This situation has put a big spotlight on “the prompt”: instruction passed to generative AI.
Behind these generative AIs is an LLM (Large Language Model) algorithm that is at the heart of the “creation”. The curious soon discovered that it was possible to channel the generative tool by passing it an instruction, in the form of simple text in natural language: this is the prompt. The subject exploded, like a miracle cure for hallucinations. It has even become a “prompt engineer” profession, which is widely advertised on recruitment sites as the perfect job: high demand, popular sector and no need for training, you just need to be able to speak and imagine how to restrict the system..
Of course, you can imagine that the mirage effect only lasts for a short time, because it’s not enough: writing a prompt is no guarantee of quality responses. We recently experienced an example with a client in the hospital sector. We had been working on recommending (or not) screening for dyslipidaemia and wanted the AI to suggest screening from the age of 40 for men and 50 for women. But this was impossible! By working solely on the prompt, we were never able to control the AI, which preferred to base itself on its training data (which came from the WHO recommendations, which recommend screening everyone from the age of 20).
Controlling a subject (and his hallucinations) by means of a prompt is therefore no more than a band-aid: it’s a hyper-specific and temporary bandage to a problem, and only addresses it in a localised way: which means that it’s neither deployable on a large scale, nor reproducible, and even less industrialisable.
The subject is a difficult one, and is occupying a number of research teams who are trying to build new prediction architectures and methods that are more reliable than prompt-engineering. Despite all these efforts, it is still impossible to guarantee that an answer provided by an AI based on an LLM is 100% correct. ChatGPT takes precautions against this with a permanent warning on its website during use.
“ChatGPT can make mistakes. Consider checking important information”.

So what should you do if you want to use this type of technology in your business?
Deeplink and its “anti-hallucination” engine
Setting up a ChatGPT-style AI engine running on selected data is becoming more commonplace and is starting to be within the reach of a keen engineer. This approach will produce a system that responds well 60% of the time. It is the increase in this ratio that very quickly becomes a headache requiring real know-how.
At Deeplink, we have decided to focus on technical and functional means to limit these undesirable effects, based on our experience with a large number of projects.
First and foremost, we work exclusively with controlled source data owned by our customers, which we prepare to reduce controllable inaccuracies. This includes the structure of the data, its selection method and numerous cleaning filters. Unlike ChatGPT, this is already a step forward.
We also chose to use a more specialised LLM engine, which runs on our servers. As well as guaranteeing data security and sovereignty, this gives us better control over the results than using an overly broad model like ChatGPT.
Finally, Deeplink has developed an anti-hallucination engine to complement its Artificial Intelligence. This technological layer reduces the perimeter of knowledge, displays the sources used to formulate the answer and evaluates the AI’s ‘sayings’ for readjustment.
Unfortunately, technology doesn’t cover everything
More than ever when a new technology is introduced, it is crucial to take the time to understand how it works, to warn the end customer of its limitations, and to work with partners who have experience in the field.
This is the mission that Deeplink has set itself by offering a solution that guarantees :
- Protection and sovereignty of its customers’ data, as there are no external links and all the algorithms (LLM, translation, etc.) run on our servers in Switzerland
- Transparency through the naming of sources
- Confidence in the response provided thanks to its anti-hallucination engine.
However, we are aware that this type of “hallucination” cannot be 100% prevented, so we encourage our customers to specify that the answers are generated by a robot, that they only represent an extract of information and that the truth is to be found in the original documents. And even if, in the event of a problem, criminal liability is more complex, as Nicolas Capt and Alexandre Jotterand point out in the ICT article, this at least helps to avoid misleading the user.
Finally, our experience also shows us that we shouldn’t forget that the reliability of an AI is often a reflection of the interaction between the human and the machine, and that if the request is ambiguous, the response will be just as imprecise. But that’s the subject of a future article 😊